Single-image super-resolution network based on dual learning strategy
Single-image super-resolution network based on dual learning strategy
1. Wuhan Research Institute of Posts & Telecommunications, Wuhan 430074, China
2. Nanjing Fiberhome Tiandi Communication Technology Co. , Ltd. , Nanjing 210019, China
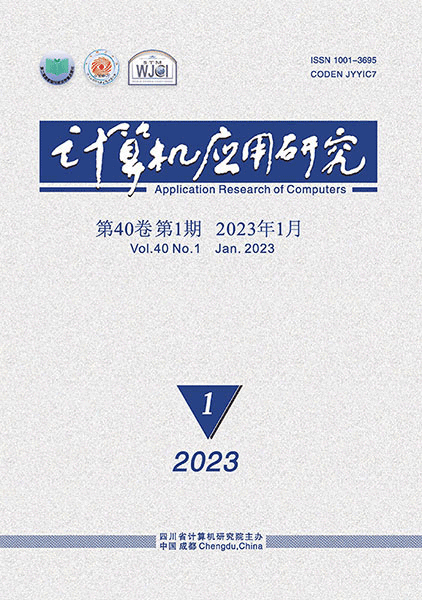
摘要
Aiming at the ill-posedness of the single-image low-resolution to super-resolution mapping, the low utilization of feature space information by the super-resolution reconstruction network, and the excessive amount of network parameters, this paper proposed a dual learning algorithm based on progressive up-sampling, which was applied to super-resolution reconstruction of a single image. The algorithm adopted deep separable convolution to significantly reduced the amount of network parameters, and constructed the progressive up-sampling network based on sub-pixel convolution to efficiently use the spatial information of the feature image. Meanwhile, it used dual learning to construct a closed-loop feedback connection network to obtain the optimal mapping function to estimate the down-sampling kernel to reconstruct low-resolution images. Analyzing on benchmark datasets such as Set5, Set14, BSDS100, Urban100, and Manga109 compared with the state-of-the-arts models, this algorithm can reduce the number of parameters by 9%, and can effectively alleviate the image edge distortion and artifact phenomenon under the large factors, the average PSRN/SSIM are 26.90/0.751、24.84/0.645、24.74/0.619、22.30/0.560、24.38/0.706.
关键词
基金项目
国家重点研发计划资助项目(2017YFB1400704)
出版信息
DOI: 10.19734/j.issn.1001-3695.2020.07.0267
出版期卷: 《计算机应用研究》 Printed Article, 2021年第38卷 第7期
所属栏目: Technology of Graphic & Image
出版页码: 2235-2240
文章编号: 1001-3695(2021)07-060-2235-06
发布历史
引用本文
陈金玲, 彭艳兵, 李念. 基于对偶学习策略的单图像超分辨率重建网络 [J]. 计算机应用研究, 2021, 38 (7): 2235-2240. (Chen Jinling, Peng Yanbing, Li Nian. Single-image super-resolution network based on dual learning strategy [J]. Application Research of Computers, 2021, 38 (7): 2235-2240. )
关于期刊
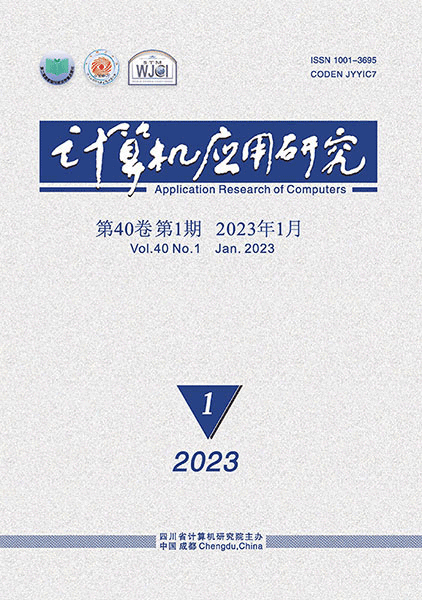
- 计算机应用研究 月刊
- Application Research of Computers
-
刊号
ISSN 1001-3695
CN 51-1196/TP
《计算机应用研究》创刊于1984年,是由四川省科技厅所属四川省计算机研究院主办的计算技术类学术刊物。
《计算机应用研究》瞄准本学科领域迫切需要的前沿技术,及时反映国内外计算机应用研究的主流技术、热点技术及最新发展趋势。主要刊载内容包括本学科领域高水平的学术论文、本学科最新科研成果和重大应用成果。栏目内容涉及计算机学科新理论、计算机基础理论、算法理论研究、算法设计与分析、区块链技术、系统软件与软件工程技术、模式识别与人工智能、体系结构、先进计算、并行处理、数据库技术、计算机网络与通信技术、信息安全技术、计算机图像图形学及其最新热点应用技术。
《计算机应用研究》拥有众多高层次读者、作者,读者对象主要为从事计算机学科领域高、中级研究人员及工程技术人员,各高等院校计算机专业及相关专业的师生。多年来《计算机应用研究》的总被引频次及Web下载率一直名列本学科同类学术刊物前茅,所刊发的学术论文以其新颖性、学术性、前瞻性、导向性、实用性而备受广大读者的喜爱。
收录和评价
- 第二届国家期刊奖百种重点期刊
- 中国期刊方阵双效期刊
- 全国中文核心期刊(北大2023年版)
- 中国科技核心期刊
- 中国科学引文数据库(CSCD)来源期刊
- RCCSE中国核心学术期刊
- 中国计算机学会会刊
- 2020—2022年科技期刊世界影响力指数(WJCI)报告收录期刊
- 中国科技期刊精品数据库全文来源期刊
- 中国学术期刊综合评价数据库来源期刊
- 《中国期刊网》《中国学术期刊(光盘版)》来源期刊
- 2017—2019年中国国际影响力优秀学术期刊(自然科学与工程技术)
- 中国精品科技期刊顶尖学术论文(F5000)项目来源期刊
- 《中国工程技术电子信息网》《电子科技文献数据库》来源期刊
- 英国《科学文摘》(INSPEC)来源期刊
- 《日本科学技术振兴机构数据库》(JST)来源期刊
- 俄罗斯《文摘杂志》(AJ, VINITI)来源期刊
- 美国《艾博思科学术数据库》(EBSCO)全文来源期刊
- 美国《剑桥科学文摘(自然科学)》(CSA(NS))核心期刊
- 波兰《哥白尼索引》(IC)来源期刊
- 美国《乌利希期刊指南(网络版)》(Ulrichsweb)收录期刊