梯度隐藏的安全聚类与隐私保护联邦学习
Gradient-hiding secure clustering and privacy-preserving federated learning
河南师范大学 a. 计算机与信息工程学院; b. 河南省教育人工智能与个性化学习重点实验室, 河南 新乡 453007
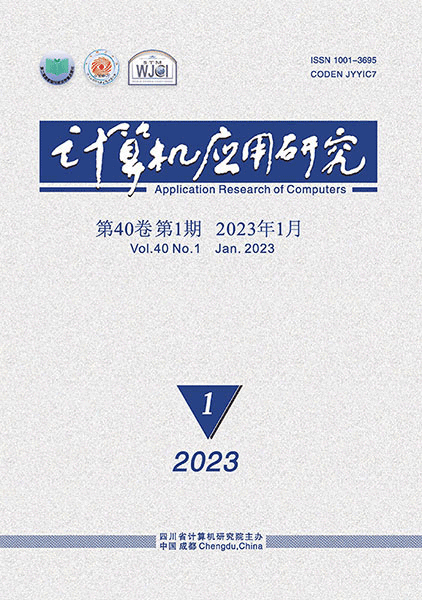
摘要
联邦学习是一种前沿的分布式机器学习算法,它在保障用户对数据控制权的同时实现了多方协同训练。然而,现有的联邦学习算法在处理Non-IID数据、梯度信息泄露和动态用户离线等方面存在诸多问题。为了解决这些问题,基于四元数、零共享与秘密共享等技术,提出了一种梯度隐藏的安全聚类与隐私保护联邦学习SCFL。首先,借助四元数旋转技术隐藏首轮模型梯度,并且在确保梯度特征分布不变的情况下实现安全的聚类分层,从而解决Non-IID数据导致的性能下降问题;其次,设计了一种链式零共享算法,采用单掩码策略保护用户模型梯度;然后,通过门限秘密共享来提升对用户离线情况的鲁棒性。与其他现有算法进行多维度比较表明,SCFL在Non-IID数据分布下准确度提高3.13%~16.03%,整体运行时间提高3~6倍。同时,任何阶段均能保证信息传输的安全性,满足了精确性、安全性和高效性的设计目标。
基金项目
河南省科技攻关计划资助项目(232102211057)
出版信息
DOI: 10.19734/j.issn.1001-3695.2023.09.0403
出版期卷: 《计算机应用研究》 印刷出版, 2024年第41卷 第6期
所属栏目: 信息安全技术
出版页码: 1851-1861
文章编号: 1001-3695(2024)06-037-1851-11
发布历史
[2023-11-16] 优先出版
[2024-06-05] 印刷出版
引用本文
李功丽, 马婧雯, 范云. 梯度隐藏的安全聚类与隐私保护联邦学习 [J]. 计算机应用研究, 2024, 41 (6): 1851-1861. (Li Gongli, Ma Jingwen, Fan Yun. Gradient-hiding secure clustering and privacy-preserving federated learning [J]. Application Research of Computers, 2024, 41 (6): 1851-1861. )
关于期刊
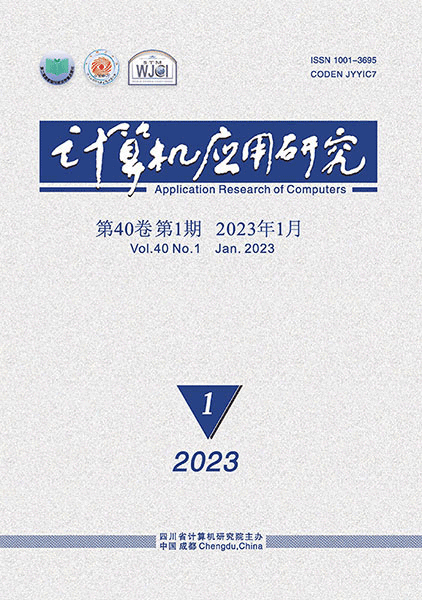
- 计算机应用研究 月刊
- Application Research of Computers
-
刊号
ISSN 1001-3695
CN 51-1196/TP
《计算机应用研究》创刊于1984年,是由四川省科技厅所属四川省计算机研究院主办的计算技术类学术刊物。
《计算机应用研究》瞄准本学科领域迫切需要的前沿技术,及时反映国内外计算机应用研究的主流技术、热点技术及最新发展趋势。主要刊载内容包括本学科领域高水平的学术论文、本学科最新科研成果和重大应用成果。栏目内容涉及计算机学科新理论、计算机基础理论、算法理论研究、算法设计与分析、区块链技术、系统软件与软件工程技术、模式识别与人工智能、体系结构、先进计算、并行处理、数据库技术、计算机网络与通信技术、信息安全技术、计算机图像图形学及其最新热点应用技术。
《计算机应用研究》拥有众多高层次读者、作者,读者对象主要为从事计算机学科领域高、中级研究人员及工程技术人员,各高等院校计算机专业及相关专业的师生。多年来《计算机应用研究》的总被引频次及Web下载率一直名列本学科同类学术刊物前茅,所刊发的学术论文以其新颖性、学术性、前瞻性、导向性、实用性而备受广大读者的喜爱。
收录和评价
- 第二届国家期刊奖百种重点期刊
- 中国期刊方阵双效期刊
- 全国中文核心期刊(北大2023年版)
- 中国科技核心期刊
- 中国科学引文数据库(CSCD)来源期刊
- RCCSE中国核心学术期刊
- 中国计算机学会会刊
- 2020—2022年科技期刊世界影响力指数(WJCI)报告收录期刊
- 中国科技期刊精品数据库全文来源期刊
- 中国学术期刊综合评价数据库来源期刊
- 《中国期刊网》《中国学术期刊(光盘版)》来源期刊
- 2017—2019年中国国际影响力优秀学术期刊(自然科学与工程技术)
- 中国精品科技期刊顶尖学术论文(F5000)项目来源期刊
- 《中国工程技术电子信息网》《电子科技文献数据库》来源期刊
- 英国《科学文摘》(INSPEC)来源期刊
- 《日本科学技术振兴机构数据库》(JST)来源期刊
- 俄罗斯《文摘杂志》(AJ, VINITI)来源期刊
- 美国《艾博思科学术数据库》(EBSCO)全文来源期刊
- 美国《剑桥科学文摘(自然科学)》(CSA(NS))核心期刊
- 波兰《哥白尼索引》(IC)来源期刊
- 美国《乌利希期刊指南(网络版)》(Ulrichsweb)收录期刊