Efficient federated learning: norm-weighted aggregation algorithm
Efficient federated learning: norm-weighted aggregation algorithm
a. School of Computer Science, b. Laboratory of Machine Learning, Southwest Petroleum University, Chengdu 610500, China
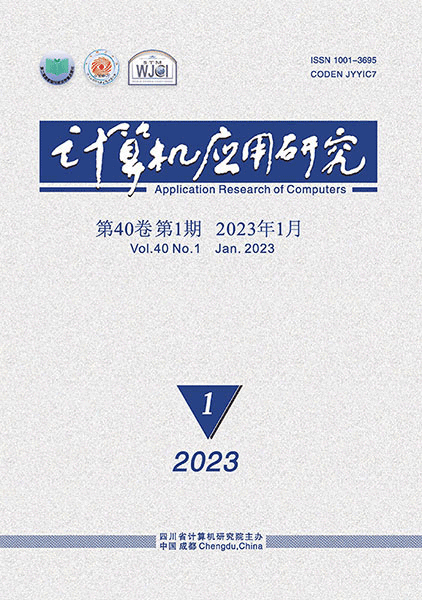
摘要
In federated learning, the non-independent and identically distributed(non-IID) data across clients leads to slower convergence of the global model and significantly increases communication costs. Existing methods collect information about the label distribution of clients to determine aggregation weights for local models, accelerating convergence, but this may leak clients' privacy. To address the slower convergence caused by non-IID data without leaking clients' privacy, this paper proposed the FedNA aggregation algorithm. FedNA achieved this goal in two ways. Firstly, it assigned aggregation weights based on the L1 norm of the class weight updates of local models to retain their contributions. Secondly, it set the class weight updates corresponding to missing classes at the clients to 0 to mitigate their impact on aggregation. Experiments were conducted under four different data distributions on two datasets. The results show that compared to FedAvg, the FedNA algorithm can reduce the number of iterations required to reach steady state by 890 at best, lowering communication costs by 44.5%. FedNA maintains clients' privacy while accelerating the convergence of the global model and decreasing communication costs. It is suitable for situations that need to protect clients privacy and are sensitive to communication efficiency.
基金项目
国家自然科学基金资助项目(61902328)
南充市科技局应用基础研究项目(SXHZ040,SXHZ051)
出版信息
DOI: 10.19734/j.issn.1001-3695.2023.07.0327
出版期卷: 《计算机应用研究》 Printed Article, 2024年第41卷 第3期
所属栏目: Special Topics in Federated Learning
出版页码: 694-699
文章编号: 1001-3695(2024)03-008-0694-06
发布历史
引用本文
陈攀, 张恒汝, 闵帆. 高效联邦学习:范数加权聚合算法 [J]. 计算机应用研究, 2024, 41 (3): 694-699. (Chen Pan, Zhang Hengru, Min Fan. Efficient federated learning: norm-weighted aggregation algorithm [J]. Application Research of Computers, 2024, 41 (3): 694-699. )
关于期刊
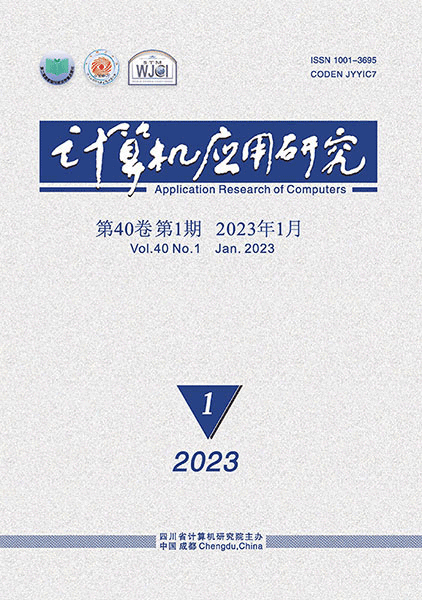
- 计算机应用研究 月刊
- Application Research of Computers
-
刊号
ISSN 1001-3695
CN 51-1196/TP
《计算机应用研究》创刊于1984年,是由四川省科技厅所属四川省计算机研究院主办的计算技术类学术刊物。
《计算机应用研究》瞄准本学科领域迫切需要的前沿技术,及时反映国内外计算机应用研究的主流技术、热点技术及最新发展趋势。主要刊载内容包括本学科领域高水平的学术论文、本学科最新科研成果和重大应用成果。栏目内容涉及计算机学科新理论、计算机基础理论、算法理论研究、算法设计与分析、区块链技术、系统软件与软件工程技术、模式识别与人工智能、体系结构、先进计算、并行处理、数据库技术、计算机网络与通信技术、信息安全技术、计算机图像图形学及其最新热点应用技术。
《计算机应用研究》拥有众多高层次读者、作者,读者对象主要为从事计算机学科领域高、中级研究人员及工程技术人员,各高等院校计算机专业及相关专业的师生。多年来《计算机应用研究》的总被引频次及Web下载率一直名列本学科同类学术刊物前茅,所刊发的学术论文以其新颖性、学术性、前瞻性、导向性、实用性而备受广大读者的喜爱。
收录和评价
- 第二届国家期刊奖百种重点期刊
- 中国期刊方阵双效期刊
- 全国中文核心期刊(北大2023年版)
- 中国科技核心期刊
- 中国科学引文数据库(CSCD)来源期刊
- RCCSE中国核心学术期刊
- 中国计算机学会会刊
- 2020—2022年科技期刊世界影响力指数(WJCI)报告收录期刊
- 中国科技期刊精品数据库全文来源期刊
- 中国学术期刊综合评价数据库来源期刊
- 《中国期刊网》《中国学术期刊(光盘版)》来源期刊
- 2017—2019年中国国际影响力优秀学术期刊(自然科学与工程技术)
- 中国精品科技期刊顶尖学术论文(F5000)项目来源期刊
- 《中国工程技术电子信息网》《电子科技文献数据库》来源期刊
- 英国《科学文摘》(INSPEC)来源期刊
- 《日本科学技术振兴机构数据库》(JST)来源期刊
- 俄罗斯《文摘杂志》(AJ, VINITI)来源期刊
- 美国《艾博思科学术数据库》(EBSCO)全文来源期刊
- 美国《剑桥科学文摘(自然科学)》(CSA(NS))核心期刊
- 波兰《哥白尼索引》(IC)来源期刊
- 美国《乌利希期刊指南(网络版)》(Ulrichsweb)收录期刊