Special Topics in Data Analysis and Knowledge Discovery
|
375-380
Interpretive subgraph generation model for knowledge graph link prediction task
Interpretive subgraph generation model for knowledge graph link prediction task
Rocket Force University of Engineering, Xi'an 710025, China
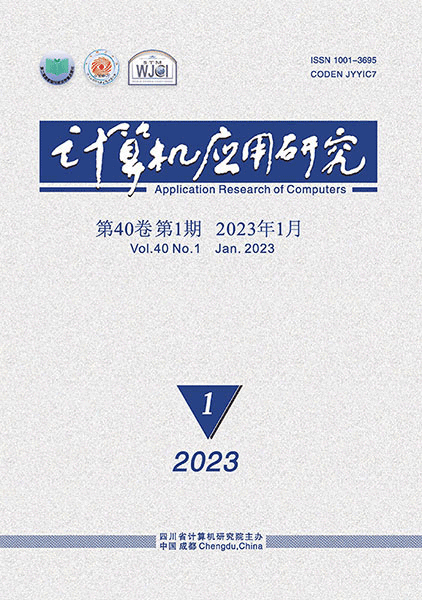
摘要
In recent years, GNN has developed rapidly, and the performance of related models in knowledge graph link prediction tasks has been significantly improved. To explain the performance improvement, the researchers need to extract the subgraph patterns learned by the GNN. However, the interpretation accuracy of existing GNN interpreters in typical multi-relational graph data scenarios such as knowledge graphs has not been verified, and related tools have not yet been implemented, resulting in difficulty in extracting interpretation subgraphs. In response to this problem, this paper proposed a knowledge graph link prediction model that converted a multi-relational knowledge graph into a uni-relational graph. The model combined the entities in the knowledge graph into new nodes and the relationship as a new node. Node features generated a new graph with only a single relationship, and trained a denoising autoencoder on the new graph to obtain link prediction capabilities, and finally used a GNN interpreter to generate subgraph explanations. Experiments on three benchmark datasets show that the relative AUC index of the link prediction model based on single-relational transformation is significantly improved compared with GraIL without transformation. Finally, this paper selected the FB15K-237 dataset to conduct explanatory subgraph extraction experiments, and verified the effectiveness of the model in directly extracting link prediction explanations.
关键词
基金项目
国家自然科学基金资助项目
陕西省科技创新团队项目
出版信息
DOI: 10.19734/j.issn.1001-3695.2023.06.0260
出版期卷: 《计算机应用研究》 Printed Article, 2024年第41卷 第2期
所属栏目: Special Topics in Data Analysis and Knowledge Discovery
出版页码: 375-380
文章编号: 1001-3695(2024)02-008-0375-06
发布历史
引用本文
姚俊萍, 袁聪, 李晓军, 等. 面向知识图谱链接预测任务的解释子图生成模型 [J]. 计算机应用研究, 2024, 41 (2): 375-380. (Yao Junping, Yuan Cong, Li Xiaojun, et al. Interpretive subgraph generation model for knowledge graph link prediction task [J]. Application Research of Computers, 2024, 41 (2): 375-380. )
关于期刊
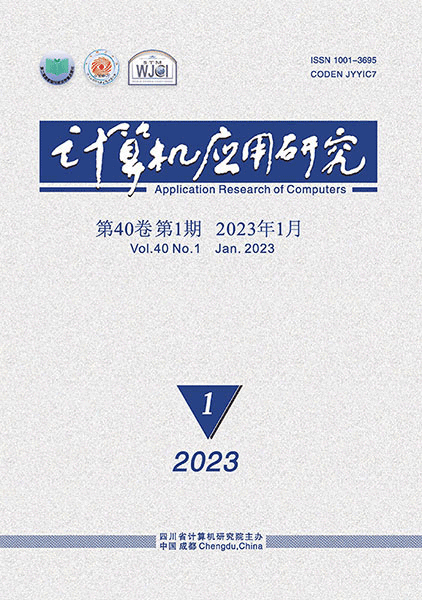
- 计算机应用研究 月刊
- Application Research of Computers
-
刊号
ISSN 1001-3695
CN 51-1196/TP
《计算机应用研究》创刊于1984年,是由四川省科技厅所属四川省计算机研究院主办的计算技术类学术刊物。
《计算机应用研究》瞄准本学科领域迫切需要的前沿技术,及时反映国内外计算机应用研究的主流技术、热点技术及最新发展趋势。主要刊载内容包括本学科领域高水平的学术论文、本学科最新科研成果和重大应用成果。栏目内容涉及计算机学科新理论、计算机基础理论、算法理论研究、算法设计与分析、区块链技术、系统软件与软件工程技术、模式识别与人工智能、体系结构、先进计算、并行处理、数据库技术、计算机网络与通信技术、信息安全技术、计算机图像图形学及其最新热点应用技术。
《计算机应用研究》拥有众多高层次读者、作者,读者对象主要为从事计算机学科领域高、中级研究人员及工程技术人员,各高等院校计算机专业及相关专业的师生。多年来《计算机应用研究》的总被引频次及Web下载率一直名列本学科同类学术刊物前茅,所刊发的学术论文以其新颖性、学术性、前瞻性、导向性、实用性而备受广大读者的喜爱。
收录和评价
- 第二届国家期刊奖百种重点期刊
- 中国期刊方阵双效期刊
- 全国中文核心期刊(北大2023年版)
- 中国科技核心期刊
- 中国科学引文数据库(CSCD)来源期刊
- RCCSE中国核心学术期刊
- 中国计算机学会会刊
- 2020—2022年科技期刊世界影响力指数(WJCI)报告收录期刊
- 中国科技期刊精品数据库全文来源期刊
- 中国学术期刊综合评价数据库来源期刊
- 《中国期刊网》《中国学术期刊(光盘版)》来源期刊
- 2017—2019年中国国际影响力优秀学术期刊(自然科学与工程技术)
- 中国精品科技期刊顶尖学术论文(F5000)项目来源期刊
- 《中国工程技术电子信息网》《电子科技文献数据库》来源期刊
- 英国《科学文摘》(INSPEC)来源期刊
- 《日本科学技术振兴机构数据库》(JST)来源期刊
- 俄罗斯《文摘杂志》(AJ, VINITI)来源期刊
- 美国《艾博思科学术数据库》(EBSCO)全文来源期刊
- 美国《剑桥科学文摘(自然科学)》(CSA(NS))核心期刊
- 波兰《哥白尼索引》(IC)来源期刊
- 美国《乌利希期刊指南(网络版)》(Ulrichsweb)收录期刊